Annotate audio in realtime#
Setup#
Test the latency of realtime annotations on a GPU (if you have one) or a CPU.
Disabling eager execution is crucial for low latency annotation - eager execution increases latencies 6-fold, from 5 ms to 30 ms.
import os
import tensorflow as tf
USE_GPU = False #
# disable existing GPUs if testing CPU
os.environ['CUDA_VISIBLE_DEVICES'] = '0' if USE_GPU else '-1'
# disabling eager execution in tensorflow speeds up annotations by ~6x
try:
from tensorflow.python.framework.ops import disable_eager_execution
disable_eager_execution()
tf.compat.v1.disable_eager_execution()
physical_devices = tf.config.list_physical_devices('GPU')
print('GPUs:', physical_devices)
physical_devices = tf.config.list_physical_devices('CPU')
print('CPUs:', physical_devices)
except Exception as e:
print(e)
GPUs: []
CPUs: [PhysicalDevice(name='/physical_device:CPU:0', device_type='CPU')]
%config InlineBackend.figure_format = 'jpg' # smaller mem footprint for page
import numpy as np
import das.utils
import das.predict
import scipy.signal
import matplotlib.pyplot as plt
import scipy.io.wavfile
import time
import subprocess
plt.style.use('ncb.mplstyle')
Load the model and runtime parameters#
model_filename = 'models/dmel_single_rt/20200430_201821'
model, params = das.utils.load_model_and_params(model_filename)
pulse_index = params['class_names'].index('pulse')
sine_index = params['class_names'].index('sine')
samplerate = params['samplerate_x_Hz']
interval = params['nb_hist'] / samplerate
print(f"Model expects input shape {model.input_shape[1]} samples and {model.input_shape[2]} channel(s).")
/Users/clemens10/miniconda3/lib/python3.7/site-packages/tensorflow/python/keras/layers/core.py:1061: UserWarning: dss.tcn.tcn is not loaded, but a Lambda layer uses it. It may cause errors.
, UserWarning)
Model expects input shape 256 samples and 1 channel(s).
Load a recording#
Samplerates of the recording and of the data the model was trained with should match. Otherwise resample the recording to match the model using scipy.signal.resample_poly(x=recording, up=int(recording_samplerate), down=int(samplerate), axis=0)
recording_samplerate, recording = scipy.io.wavfile.read('dat/dmel_song_rt.wav')
recording = np.atleast_2d(recording).T
plt.figure(figsize=(30, 4))
plt.plot(recording[:100_000], c='k') # plot the first 10 seconds
plt.show()
print(f"Sample rate of the recording ({recording_samplerate}Hz), sample rate expected by the model ({samplerate}Hz). Both should match.")

Sample rate of the recording (10000Hz), sample rate expected by the model (10000Hz). Both should match.
Define a virtual microphone for testing#
To demonstrate real-time inference, we create a virtual microphone that returns chunk of audio data in real time. The microphone takes a full recording and returns chunks of data at a rate determined by the chunk duration. In a real experimental setup, this would be a function that runs the data acquisition or a multi-processing queue that gets fed by the acquisition system.
def virtual_microphone(data: np.array, interval: float, samplerate: float, block: bool = True):
RUN = True
current_position = 0
t1 = time.time() - 0.025
t0 = time.time()
while RUN:
current_position += interval * samplerate
start_sample = int(current_position)
end_sample = int(current_position + interval * samplerate)
chunk = data[start_sample:end_sample]
if block:
while (t1-t0)<interval: # wait til interval has elapsed
time.sleep(0.001)
t1 = time.time()
if end_sample >= data.shape[0]:
RUN = False
yield None
else:
yield chunk
t0 = time.time()
mike = virtual_microphone(recording, interval, samplerate, block=True)
rec = next(mike)
print(f"Microphone produces {rec.shape[0]} samples and {rec.shape[1]} channel(s) per chunk.")
print('Consuming and plotting 100 chunks...')
recording_latencies = []
for _ in range(100):
with das.utils.Timer() as t:
rec = next(mike)
recording_latencies.append(t.elapsed * 1000)
plt.plot(rec)
plt.ylim(-1, 1)
plt.title('Individal chunks')
plt.xlabel('Samples')
plt.xlim(0, rec.shape[0])
plt.show()
print(f"Takes {np.median(recording_latencies):1.1f} ms to return {rec.shape[0] / samplerate * 1000:1.1f} ms of audio data.")
Microphone produces 256 samples and 1 channel(s) per chunk.
Consuming and plotting 100 chunks...
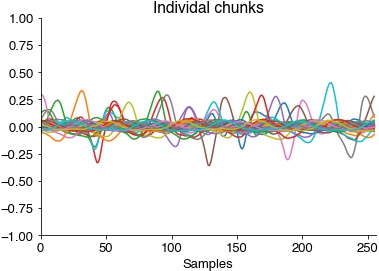
Takes 26.0 ms to return 25.6 ms of audio data.
Test real-time annotation#
Test the model and a simple song detection routine with a chunk that contains song. A specific song type is detect if the corresponding confidence exceeds 0.7 during a chunk.
def report_song(prediction, pulse_index, sine_index):
report = 'pulse? ' + ('yes' if np.max(out[:, pulse_index]) > 0.7 else ' no')
report += ' sine? ' + ('yes' if np.max(out[:, sine_index]) > 0.7 else ' no')
return report
# consume a couple of chunks until there is song
for _ in range(9):
d = next(mike)
d = d[np.newaxis, ...]
out = model.predict(d, verbose=0)
plt.subplot(211)
plt.plot(d[0, ...])
plt.ylabel('Audio [V]')
plt.subplot(212)
plt.plot(out[0])
plt.ylabel('Confidence\nscore')
plt.xlabel('Samples')
plt.legend(params['class_names'])
/Users/clemens10/miniconda3/lib/python3.7/site-packages/tensorflow/python/keras/engine/training.py:2426: UserWarning: `Model.state_updates` will be removed in a future version. This property should not be used in TensorFlow 2.0, as `updates` are applied automatically.
warnings.warn('`Model.state_updates` will be removed in a future version. '
<matplotlib.legend.Legend at 0x7ffdec78f4d0>
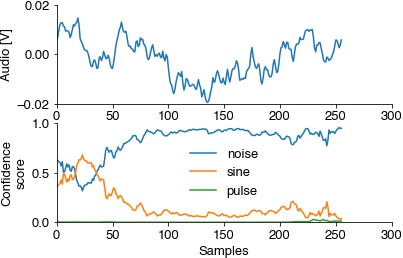
Run annotation#
Run the virtual microphone for 100 chunks to estimate annotation latencies.
latencies = []
runs = 0
mike = virtual_microphone(recording, interval, samplerate, block=True)
while runs < 100:
runs += 1
with das.utils.Timer() as dt:
d = next(mike) # get a new chunk
d = d[np.newaxis, ...]
out = model.predict(d, verbose=0) # run inference on the chunk
# detect pulse and sine
result = report_song(out[0, ...], pulse_index, sine_index)
# print(result)
latencies.append(dt.elapsed * 1000)
Evaluate latencies#
Timing on GPUs can be more variable than on CPUs since a major contributor to latency is the transfer of data from/to the GPU.
Subtract the recording latency to get an estimate of the annotation latency.
annotation_latencies = np.array(latencies) - np.median(recording_latencies)
print('Annotation latencies')
print(f' 5, 50, 95 percentiles: {np.percentile(annotation_latencies, [5, 50, 95])} ms')
print(f' min {np.min(annotation_latencies):1.2f} ms, max {np.max(annotation_latencies):1.2f} ms')
plt.figure(figsize=(5, 5))
plt.plot(np.array(annotation_latencies))
plt.ylabel('Annotation latency [ms]')
plt.xlabel('Chunk#')
plt.ylim(0, np.max(annotation_latencies))
plt.show()
Annotation latencies
5, 50, 95 percentiles: [4.6493622 5.443717 6.2767092] ms
min 4.53 ms, max 54.00 ms
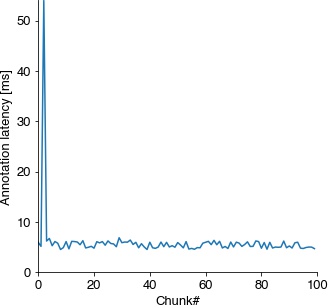